Pillars of Readiness
By now, the story is well told on how artificial intelligence is changing businesses, all the way down to impacting core business models. In 2017, Amazon bought Whole Foods then later opened their Amazon Go automated store. Since then, they’ve been using AI to understand and improve the physical retail shopping experience. In 2018, Keller Williams announced a pivot towards becoming an artificial intelligence-driven technology company to compete with the tech-centric entrants into the market like Zillow and Redfin.
These companies are not alone. According to a study by MIT Sloan Management Review, one trillion dollars of new profit will be created from the use of artificial intelligence technologies by 2030. That is roughly 10% of all total profits projected for that time. Still, most companies have yet to implement artificial intelligence in their business. Depending on what study you ready, 70%-80% of all businesses have yet to begin any AI implementation whatsoever.
The reality is most companies are just not ready for AI, and if they try before they are ready, they will fail. For AI projects to be successful, you likely need to shore up a few areas.
6 Pillars of AI Readiness:
- Culture
- Data
- Strategy
- Technology
- Expertise
- Operations
Regardless of the level of expertise in your company or ability to invest, achieving meaningful results from artificial intelligence requires six key areas to be optimal tuned for success to be achieved. Even if a small portion of the profit forecasts are true, readiness matters.
Symptoms of Readiness
If AI is the answer, what is the problem? Many companies still struggle with a general understanding of how AI can make a meaningful impact. They don’t realize there are common challenges that plague most businesses where artificial intelligence can provide solutions. Identifying these challenges are signs that your company may be ready to see benefit from artificial intelligence technologies.
Symptoms of Readiness:
- Mundane tasks prone to error
- Not enough people to get jobs done
- Need creative ways to get data
- Desire to predict trends or make better decisions
- Seeking new business models or to enter new markets
If any of these problems are relevant or a priority, AI has well documented benefits. Artificial Intelligence today is best suited to automate tasks, predict phenomenon, and even generate more data.
Leveraging AI for data generation gets less pickup. To level-set, data is the most important component of this whole equation (more on that later). We are also surrounded by data exhaust, where very little is captured and processed for meaningful intelligence. For example, computer vision and optical character recognition can be used to extract data from paper contracts or receipts to use to make future predictions.
Without a Culture of Innovation, You Are Not Ready For AI.
A company’s culture is paramount for embracing data and enhanced capabilities. Amazon, Keller Williams, Google, Facebook, and Walmart all have a track record of innovation. They have people and resources dedicated to the research and development of new ideas. Businesses must be fearless in courting innovation and not afraid to spend money for fear of failing in search of success. A willingness to embrace and invest in innovation is a must.
Along with innovation, organizations must see data as a corporate asset. The business and culture must value data and be invested in collecting it. In the future there will be far more cultural considerations that will dictate what and how AI will be adopted. Issues like privacy, explainability, and ethics will all be cultural considerations, dictating where the technology will and won’t be applied.
Without Sufficient Quantity and Quality Data, Artificial Intelligence Won’t Work.
By now it should be no surprise that data is the lifeblood of artificial intelligence. Without data, the algorithms cannot learn and make predictions. Data must be present in sufficient quantity AND quality. Mountains of data may not be enough if signal about the phenomenon you are looking to learn does not exist.
Many AI Pioneers already have robust data and analytics infrastructures along with a broad understanding of what it takes to develop the data for training AI algorithms. AI Investigators and Experimenters, by contrast, struggle because they have little analytics expertise and keep their data largely in silos, where it is difficult to integrate.
(Report: MIT Sloan Management Review)
In fact, 90% of the effort to deploy AI solutions lies in wrangling data and feature engineering. The more high-quality data, the more accurate the predictions. Bad data is the number one reason most AI projects fail.
Without a strategy, AI solutions risk never making it into production.
As stated before, the business must value data as a corporate asset. That is fundamental to your strategy. However, the thinking must go further. Any AI program must be tightly aligned to support the corporate strategy. Artificial intelligence is an enhanced capability to achieve your business goals.
Companies committed to adopting AI need to make sure their strategies are transformational and should make AI central to revising their corporate strategies.
(Report: MIT Sloan Management Review)
AI for AI’s sake often leads to long, drawn-out projects that never produce any real value. CEO support is the ideal. Executive sponsors are critical to ensure proper alignment, set business metrics for any technology implementation, and provide air cover against any disputes over data or technology involvement.
AI entrepreneur Jordan Jacobs lays out the three ingredients for a winning strategy:
Getting buy-in from the top executives and the employees who will use the system, identifying clearly the business problem to be solved, and setting metrics to demonstrate the technology’s return on investment.
(Jordan Jacobs)
According to MIT Technology Review, there are key questions a business must answer to formulate their strategy:
- What is the problem the business is trying to solve? Is this problem amenable to AI and machine learning?
- How will the AI solve the problem? How has the business problem been reframed into a machine-learning problem? What data will be needed to input into the algorithms?
- Where does the company source its data? How is the data labeled?
- How often does the company validate, test and audit its algorithms for accuracy and bias?
- Is AI, or machine learning, the best and only way to solve this problem? Do the benefits outweigh potential privacy violations and other negative impacts?
Without cloud-based technologies, many AI solutions can’t operate.
For most businesses, embracing cloud-based computing and storage technologies are critical for AI programs to produce effectively. Artificial intelligence models require tremendous compute power to process massive data sets. This requires businesses to have ready access to computer power on-demand.
Since 2012, the amount of computation used in the largest AI training runs has been increasing exponentially with a 3.5 month doubling time (by comparison, Moore’s Law had an 18 month doubling period).
(OpenAI)
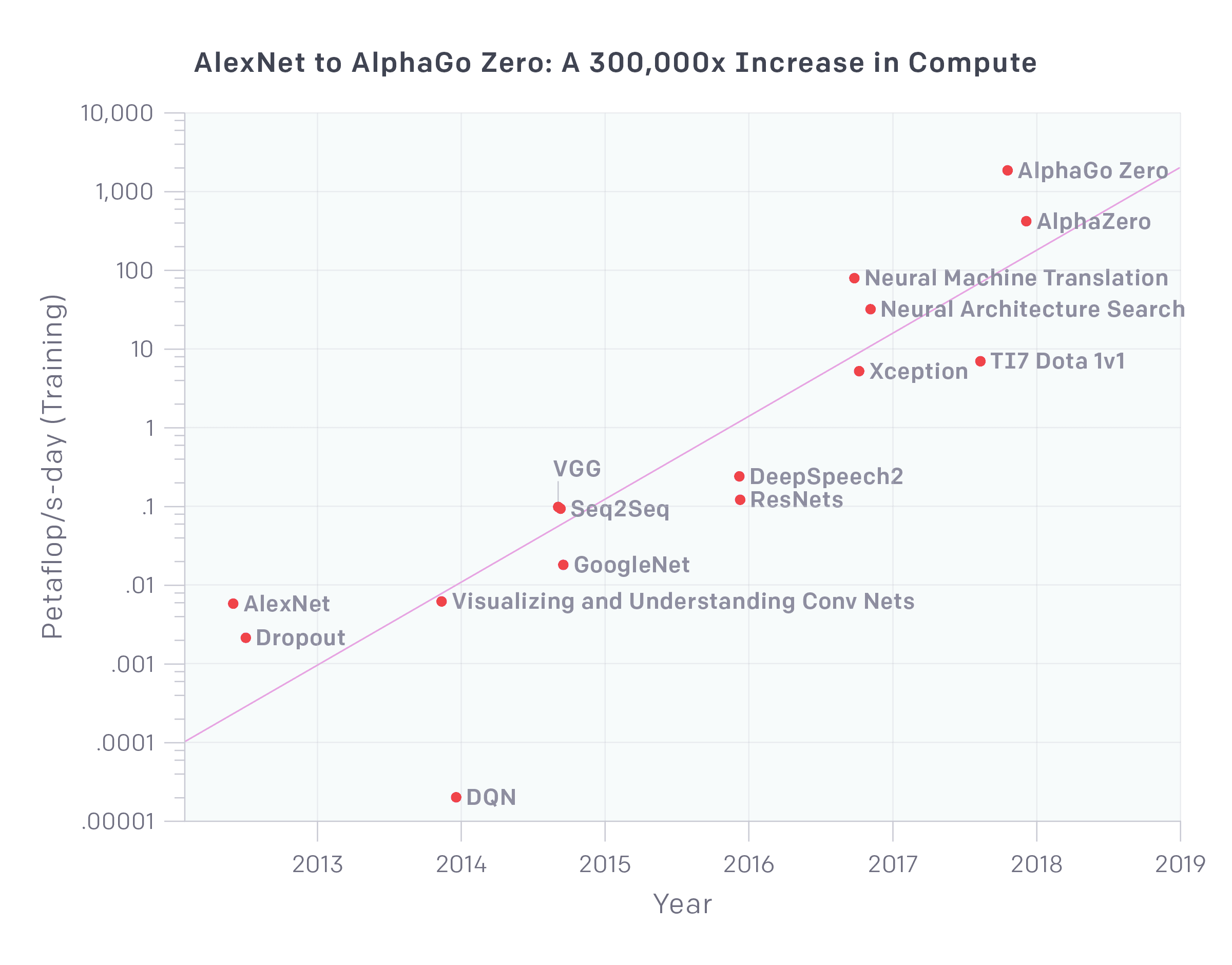
If AI programs are to be successful, companies need to embrace cloud technologies. They need to be willing to adopt platforms that provision GPU clusters based on workloads required by deployed models. Cloud is important because owning the hardware can cost over a million dollars for a single cluster, according to OpenAI.
Without internal expertise, AI adoption is challenging.
To successfully move AI projects through the development life-cycles, from data to production, you need to have in-house technical expertise. At minimum, you need to have dedicated data managers who can help wrangle data to train models. It is important to have software engineers or DevOps leads who can help move trained models into production environments so non-technical stakeholders can easily run reports. These two roles can be augmented by services providers that build and train models. However, it is better if you have data scientists, analysts, and data engineers who can help strategize and execute projects as well.
Without well-defined technology processes, projects risk never making it into production.
Another common risk associated with AI projects is that trained models don’t transition into production. It is important to have a connection between the business strategy and the delivery of AI technology. It’s especially important to have a plan in place for how to access the data, train the model, and handoff the model to be deployed as a usable solution.
Develop an operating model that defines AI roles and responsibilities for the business, technology teams, external vendors, and existing BPM centers of excellence. Some firms will separate model development — i.e., selecting and developing the AI, data science, and algorithms — from implementation. But over time, tech management will own deployment and operational management.
(Report: Forrester)
Moving forward it will be important to have documented processes for post-deployment. Once multiple models are in production you need to monitor models for performance and have a documented process for re-training.
Take an AI readiness assessment.
Many companies are not fully equipped to realize the full benefits created by artificial intelligence. And it is hard to know if “good enough” is good enough. Start by evaluating how equipped your business may be to successfully deploy projects. There are many ways to assess your readiness and de-risk investment. Online AI readiness assessments will help you start to understand if your organization has the prerequisites to successfully execute initial projects. If you are not ready, there is a lot of opportunity at stake. The most valuable thing you can do is to start to get ready. If you have executive buy-in, partner with consultancies or hire an AI strategist who can help put the pieces in place.
Originally Posted on KUNGFU.AI